In this case study we demonstrate how you can use DIAL eXpressive Logic (XL) to uncover patterns and relationships in clinical trial data using natural language, all while keeping human in the loop.
We loaded a sample clinical trial dataset of 1,000 patients into DIAL XL to investigate the hypothesis that the treatment group may influence the frequency of adverse events or study dropout in a gender-specific way. Additionally, we aimed to assess whether certain vital sign statistics, such as blood pressure and cholesterol levels, are associated with adverse events or study dropout across different treatment groups and genders.
In this case study, DIAL XL's AI agents perform several tasks based on natural language prompts from the user:
- The AI agent interprets a query from a user, identifies the relevant dataset columns (e.g., gender, treatment group), generates a worksheet with the requested data, and provides a summary of key findings.
- Further, it creates a table, grouping patients by drug and calculate adverse effect frequencies.
- The agent focuses on patients with adverse effects, generates an intermediate table, and summarizes their blood pressure readings.
- The agent constructs tables and charts to visualize patterns in dropout rates and their correlation with adverse effects.
At every step, DIAL XL provided transparency by generating the underlying formulas, explaining the processes, and offering suggestions for next steps. This allowed users to review, validate, and refine the analysis—ensuring human oversight and control.
Solutions like DIAL XL are transforming clinical data analysis by enabling researchers to uncover hidden patterns, generate actionable insights, and streamline workflows—all in a faster, more efficient, and user-friendly way. Researchers can also create reusable templates to analyze different datasets to discover trends and validate hypotheses.
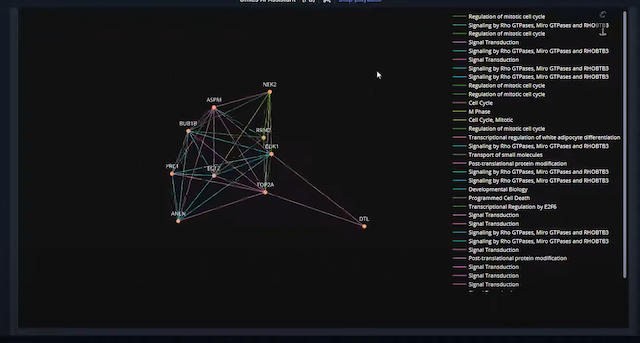
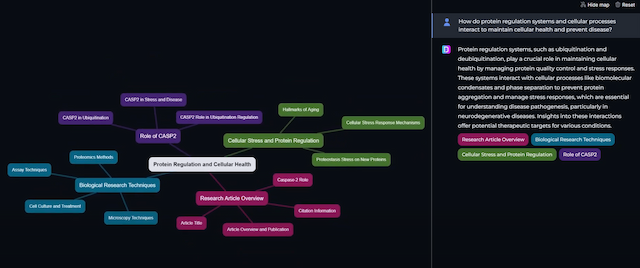